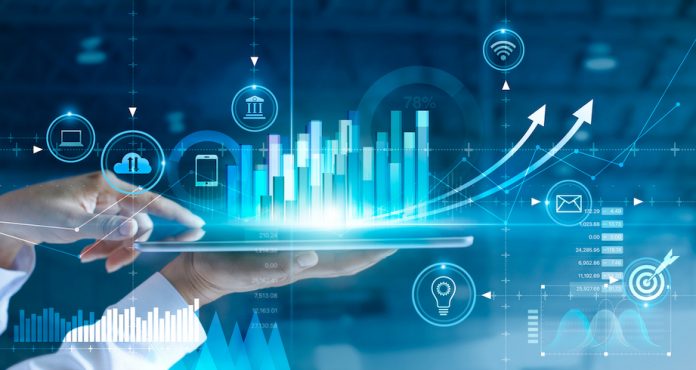
Forecasting the demand of hotel reservations is a key piece of setting strategic pricing and establishing inventory allocation practices. Managers can optimize overbooking limits, define cancellation policies, and predict net revenue as their confidence in anticipated demand increases. If cancellation policies are too strict, customers are discouraged from ever making a reservation. If overbooking limits are too high, customers may be refused service, discouraging them from ever booking with this hotel or company in the future. Accurate demand forecasting models—including an accurate predictor of cancellations—will help managers strike a balance in their booking policies, increasing overall customer satisfaction and optimizing cost models.
What makes forecasting hotel reservation cancellations difficult?
Human behavior is highly complex, and thus very challenging to model and predict. As reservation trends are driven by human behavior, his generalization holds true for the hotel industry as well. Little is known about why guests cancel, or how to encourage guests not to cancel. Data identifies a few common reasons to cancel, such as illness and bad weather, but these reasons cannot fully explain or predict cancellation trends.
The rise in popularity of internet booking makes forecasting even more challenging. On top of existing reasons to cancel (e.g., bad weather, changing plans, illness, etc.), web bookings are the easiest way to make, change, and cancel reservations. Guests can easily make a last-minute decision between several options with convenient side-by-side comparison through their online travel source. Many deal-seeking customers place multiple reservations and cancel all but one based on their perceived best value.
Even as general cancellation trends can be predicted, it’s even more difficult to pick out which specific guests might be most likely to cancel. Knowledge of which individual guests are likely to cancel is a key factor in most customer service-oriented strategies that hope to reduce the number of cancellations.
What methods have successfully predicted cancellation trends and probability?
Machine learning excels in finding patterns in large data sets. Although web booking brings many challenges to the cancellation process, it also provides a wealth of information on general trends and specific behavior patterns. As with most big data problems, there is an immense amount of complex data to sift through, providing an opportunity to extract meaning and value by introducing machine learning. To predict if an individual would cancel a reservation, one recent study examined 13 different variables tied to the booking process, including:
- Date (month and day) the reservation was made
- How far in advance the reservation was created ahead of the stay
- Start and end date (month and day) of the stay
- Length of stay (number of consecutive nights)
- Number of weekend (Saturday or Sunday) days included in the stay
- Deposit payment amount
- Nightly price
- Number of adult guests
- Booking method
These researchers applied a specialized machine learning model, called a genetic algorithm, that generated a cancellation probability for each customer by evaluating the interactions between these 13 variables. This study was particularly promising, as it used only the material available internally on a booking website—without consulting external databases or extracting users’ personal information—to successfully predict cancellation with 98 percent accuracy. The same research team tested other algorithms that were also relatively effective, including a random forest with 80 percent accuracy, a support vector machine with 75 percent accuracy, and a C 5.0 decision tree with 79 percent accuracy.
Conclusion
Machine learning and big data models can provide hotel managers with a reliable way to anticipate cancellation trends and identify the individuals that are most likely to cancel reservations. Forecasting suggests a balance between overbooking limits with cancellation policies to maximize revenue and customer satisfaction. As these models improve in speed and accuracy, their value to the hotel industry will continue to increase.